Поиск по каталогу |
(строгое соответствие)
|
- Профессиональная
- Научно-популярная
- Художественная
- Публицистика
- Детская
- Искусство
- Хобби, семья, дом
- Спорт
- Путеводители
- Блокноты, тетради, открытки
Regression with Hyperdimensional Features. Application to Chemometric calibration
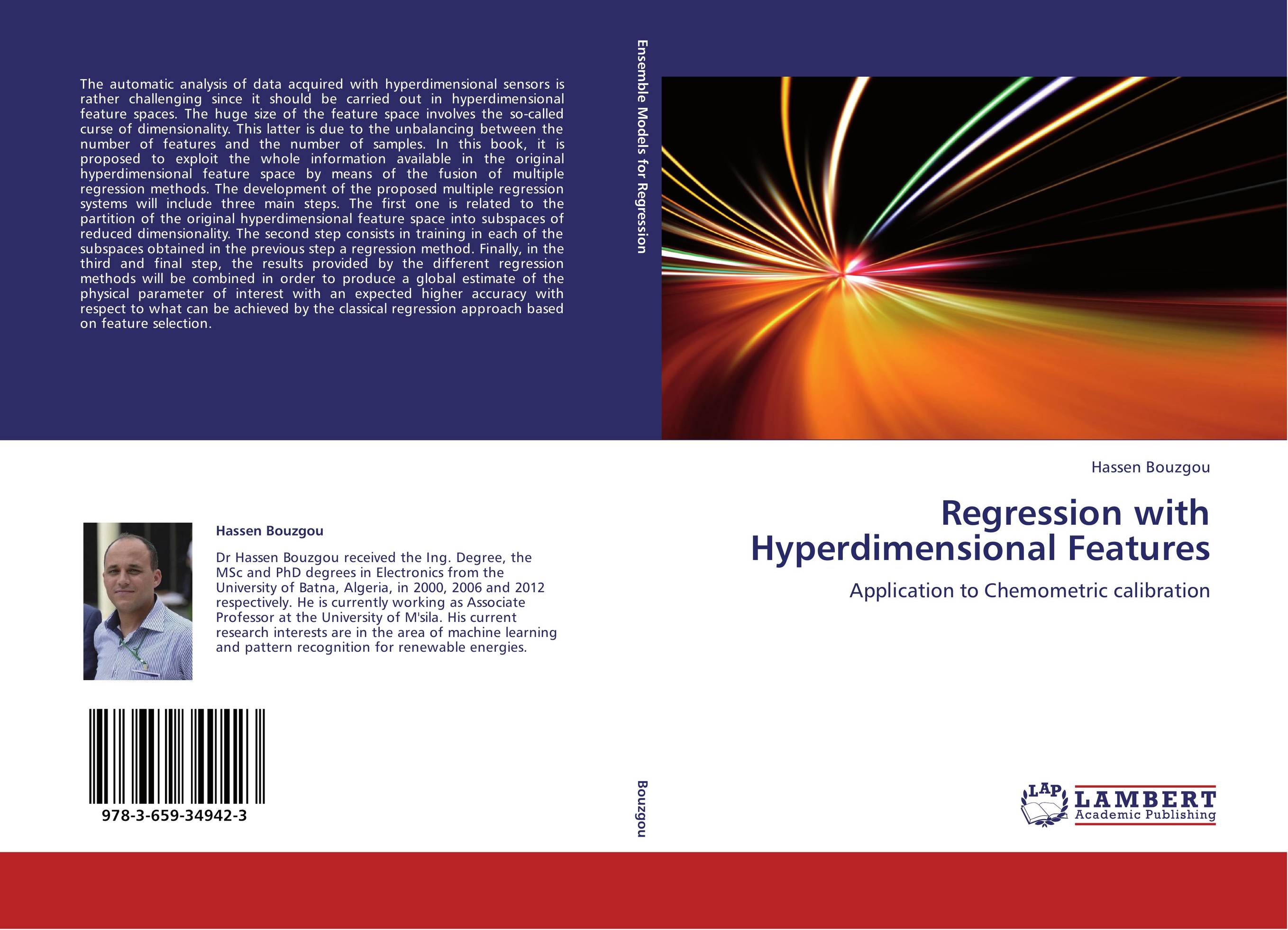
В наличии
Местонахождение: Алматы | Состояние экземпляра: новый |

Бумажная
версия
версия
Автор: Hassen Bouzgou
ISBN: 9783659349423
Год издания: 2013
Формат книги: 60×90/16 (145×215 мм)
Количество страниц: 144
Издательство: LAP LAMBERT Academic Publishing
Цена: 38058 тг
Положить в корзину
Позиции в рубрикаторе
Отрасли знаний:Код товара: 118897
Способы доставки в город Алматы * комплектация (срок до отгрузки) не более 2 рабочих дней |
Самовывоз из города Алматы (пункты самовывоза партнёра CDEK) |
Курьерская доставка CDEK из города Москва |
Доставка Почтой России из города Москва |
Аннотация: The automatic analysis of data acquired with hyperdimensional sensors is rather challenging since it should be carried out in hyperdimensional feature spaces. The huge size of the feature space involves the so-called curse of dimensionality. This latter is due to the unbalancing between the number of features and the number of samples. In this book, it is proposed to exploit the whole information available in the original hyperdimensional feature space by means of the fusion of multiple regression methods. The development of the proposed multiple regression systems will include three main steps. The first one is related to the partition of the original hyperdimensional feature space into subspaces of reduced dimensionality. The second step consists in training in each of the subspaces obtained in the previous step a regression method. Finally, in the third and final step, the results provided by the different regression methods will be combined in order to produce a global estimate of the physical parameter of interest with an expected higher accuracy with respect to what can be achieved by the classical regression approach based on feature selection.
Ключевые слова: Chemometrics; Machine learning; Pattern Recognition