Поиск по каталогу |
(строгое соответствие)
|
- Профессиональная
- Научно-популярная
- Художественная
- Публицистика
- Детская
- Искусство
- Хобби, семья, дом
- Спорт
- Путеводители
- Блокноты, тетради, открытки
Improving the Efficiency of SfM and its Applications.
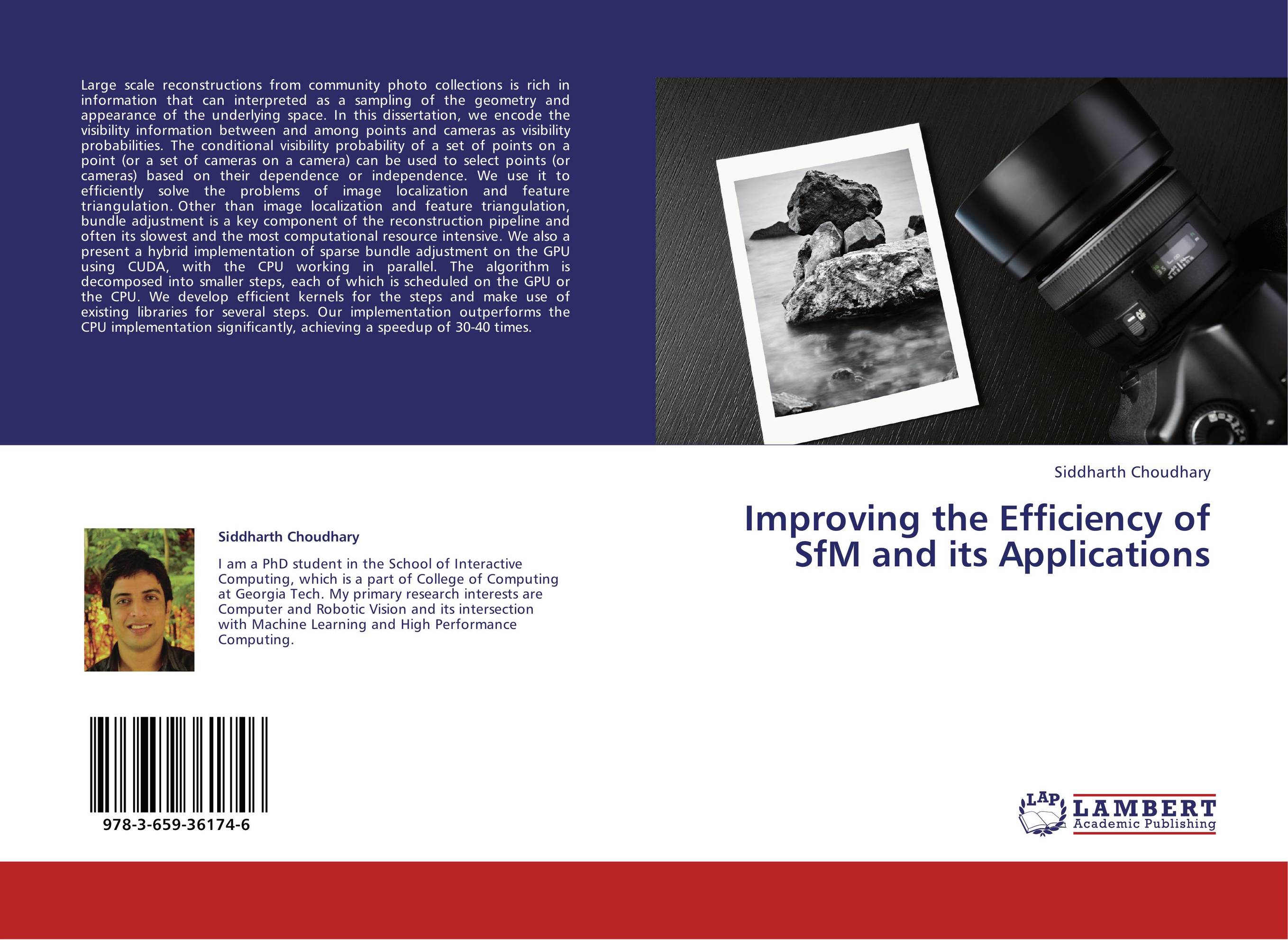
В наличии
Местонахождение: Алматы | Состояние экземпляра: новый |

Бумажная
версия
версия
Автор: Siddharth Choudhary
ISBN: 9783659361746
Год издания: 2013
Формат книги: 60×90/16 (145×215 мм)
Количество страниц: 92
Издательство: LAP LAMBERT Academic Publishing
Цена: 30926 тг
Положить в корзину
Позиции в рубрикаторе
Отрасли знаний:Код товара: 120030
Способы доставки в город Алматы * комплектация (срок до отгрузки) не более 2 рабочих дней |
Самовывоз из города Алматы (пункты самовывоза партнёра CDEK) |
Курьерская доставка CDEK из города Москва |
Доставка Почтой России из города Москва |
Аннотация: Large scale reconstructions from community photo collections is rich in information that can interpreted as a sampling of the geometry and appearance of the underlying space. In this dissertation, we encode the visibility information between and among points and cameras as visibility probabilities. The conditional visibility probability of a set of points on a point (or a set of cameras on a camera) can be used to select points (or cameras) based on their dependence or independence. We use it to ef?ciently solve the problems of image localization and feature triangulation. Other than image localization and feature triangulation, bundle adjustment is a key component of the reconstruction pipeline and often its slowest and the most computational resource intensive. We also a present a hybrid implementation of sparse bundle adjustment on the GPU using CUDA, with the CPU working in parallel. The algorithm is decomposed into smaller steps, each of which is scheduled on the GPU or the CPU. We develop ef?cient kernels for the steps and make use of existing libraries for several steps. Our implementation outperforms the CPU implementation signi?cantly, achieving a speedup of 30-40 times.
Ключевые слова: Computer Vision, 3D reconstruction, Image based Localization