Поиск по каталогу |
(строгое соответствие)
|
- Профессиональная
- Научно-популярная
- Художественная
- Публицистика
- Детская
- Искусство
- Хобби, семья, дом
- Спорт
- Путеводители
- Блокноты, тетради, открытки
Defining Stochastic Inference To Improve Pattern Recognition.
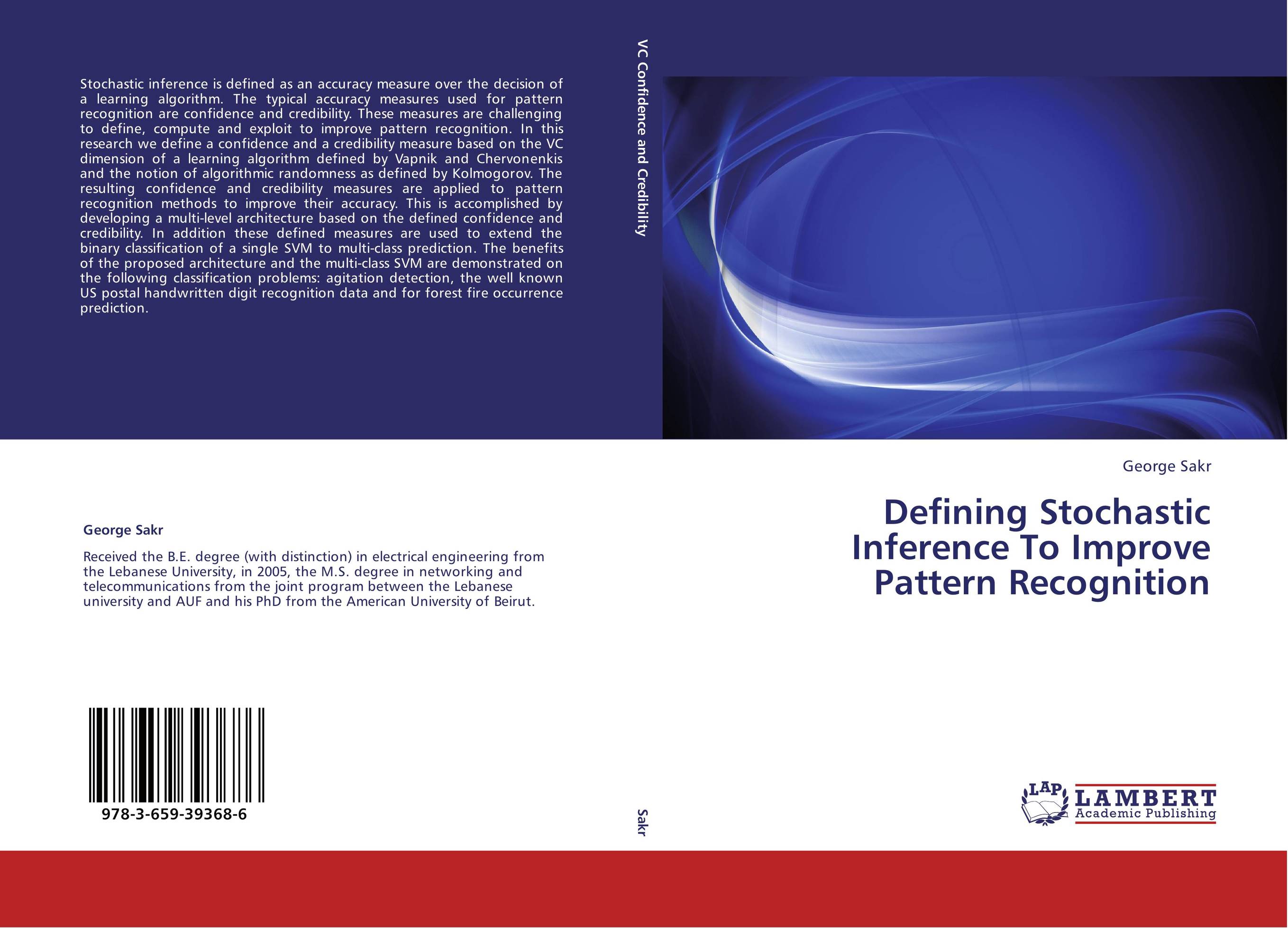
В наличии
Местонахождение: Алматы | Состояние экземпляра: новый |

Бумажная
версия
версия
Автор: George Sakr
ISBN: 9783659393686
Год издания: 2013
Формат книги: 60×90/16 (145×215 мм)
Количество страниц: 152
Издательство: LAP LAMBERT Academic Publishing
Цена: 39875 тг
Положить в корзину
Способы доставки в город Алматы * комплектация (срок до отгрузки) не более 2 рабочих дней |
Самовывоз из города Алматы (пункты самовывоза партнёра CDEK) |
Курьерская доставка CDEK из города Москва |
Доставка Почтой России из города Москва |
Аннотация: Stochastic inference is defined as an accuracy measure over the decision of a learning algorithm. The typical accuracy measures used for pattern recognition are confidence and credibility. These measures are challenging to define, compute and exploit to improve pattern recognition. In this research we define a confidence and a credibility measure based on the VC dimension of a learning algorithm defined by Vapnik and Chervonenkis and the notion of algorithmic randomness as defined by Kolmogorov. The resulting confidence and credibility measures are applied to pattern recognition methods to improve their accuracy. This is accomplished by developing a multi-level architecture based on the defined confidence and credibility. In addition these defined measures are used to extend the binary classification of a single SVM to multi-class prediction. The benefits of the proposed architecture and the multi-class SVM are demonstrated on the following classification problems: agitation detection, the well known US postal handwritten digit recognition data and for forest fire occurrence prediction.
Ключевые слова: Artificial Intelligence, Support Vector Machines, Confidence Measure