Поиск по каталогу |
(строгое соответствие)
|
- Профессиональная
- Научно-популярная
- Художественная
- Публицистика
- Детская
- Искусство
- Хобби, семья, дом
- Спорт
- Путеводители
- Блокноты, тетради, открытки
Classification of data mining techniques in intrusion detection. Classification Techniques in Data Mining
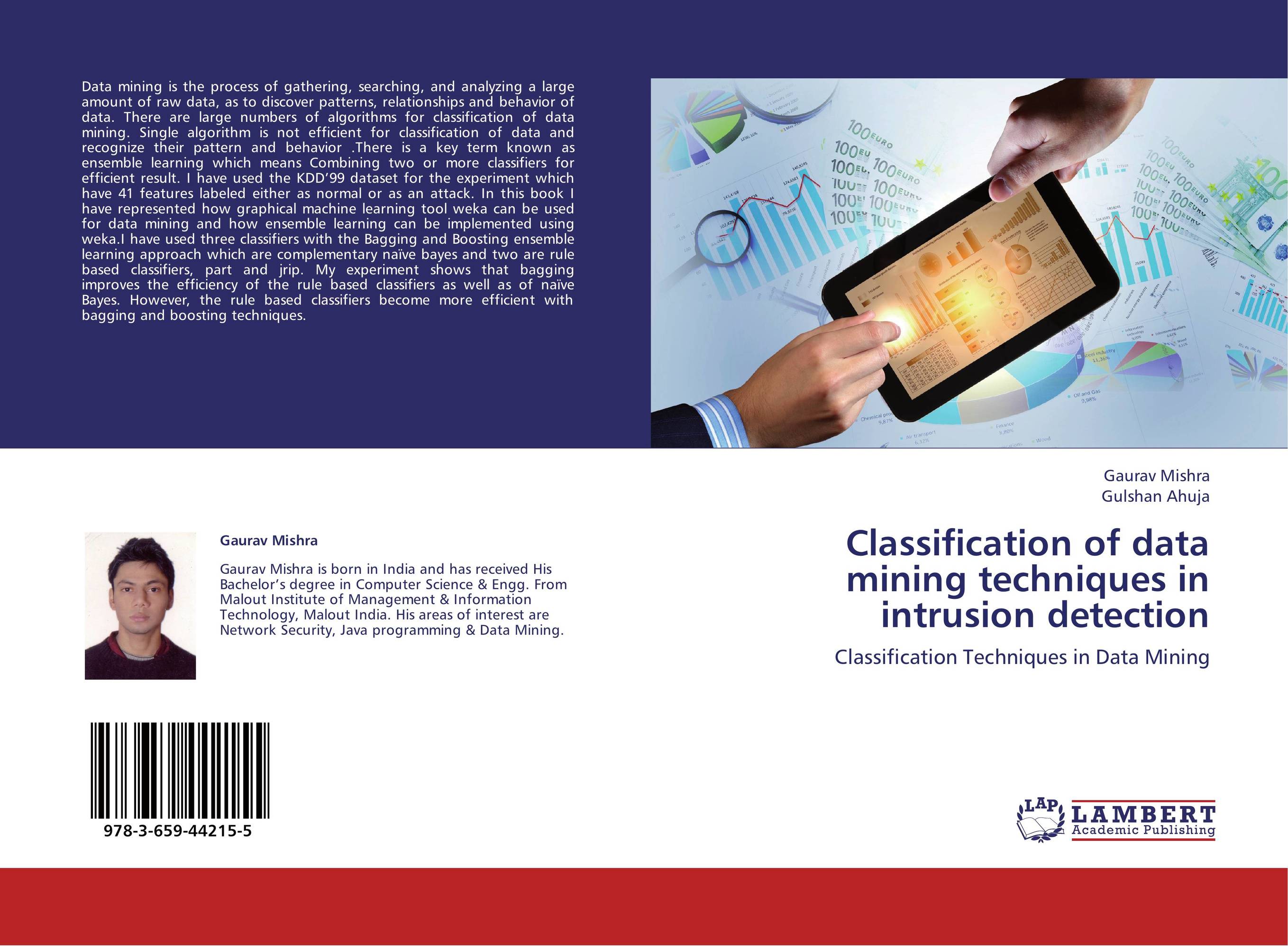
В наличии
Местонахождение: Алматы | Состояние экземпляра: новый |

Бумажная
версия
версия
Автор: Gaurav Mishra and Gulshan Ahuja
ISBN: 9783659442155
Год издания: 2013
Формат книги: 60×90/16 (145×215 мм)
Количество страниц: 52
Издательство: LAP LAMBERT Academic Publishing
Цена: 22584 тг
Положить в корзину
Позиции в рубрикаторе
Отрасли знаний:Код товара: 125286
Способы доставки в город Алматы * комплектация (срок до отгрузки) не более 2 рабочих дней |
Самовывоз из города Алматы (пункты самовывоза партнёра CDEK) |
Курьерская доставка CDEK из города Москва |
Доставка Почтой России из города Москва |
Аннотация: Data mining is the process of gathering, searching, and analyzing a large amount of raw data, as to discover patterns, relationships and behavior of data. There are large numbers of algorithms for classification of data mining. Single algorithm is not efficient for classification of data and recognize their pattern and behavior .There is a key term known as ensemble learning which means Combining two or more classifiers for efficient result. I have used the KDD’99 dataset for the experiment which have 41 features labeled either as normal or as an attack. In this book I have represented how graphical machine learning tool weka can be used for data mining and how ensemble learning can be implemented using weka.I have used three classifiers with the Bagging and Boosting ensemble learning approach which are complementary na?ve bayes and two are rule based classifiers, part and jrip. My experiment shows that bagging improves the efficiency of the rule based classifiers as well as of na?ve Bayes. However, the rule based classifiers become more efficient with bagging and boosting techniques.
Ключевые слова: Data Mining, classification, Intrusion, Weka, Naive Bayes, Naive Bayes, classification rules, denial of service attack, weka classifier, KDD Datset, jrip, bagging ensemble aproach weka java alogrithm