Поиск по каталогу |
(строгое соответствие)
|
- Профессиональная
- Научно-популярная
- Художественная
- Публицистика
- Детская
- Искусство
- Хобби, семья, дом
- Спорт
- Путеводители
- Блокноты, тетради, открытки
Efficient MCMC Estimation of Mixed Effects Binary Logit Models. Comparison of Various Markov Chain Monte Carlo Estimation Techniques
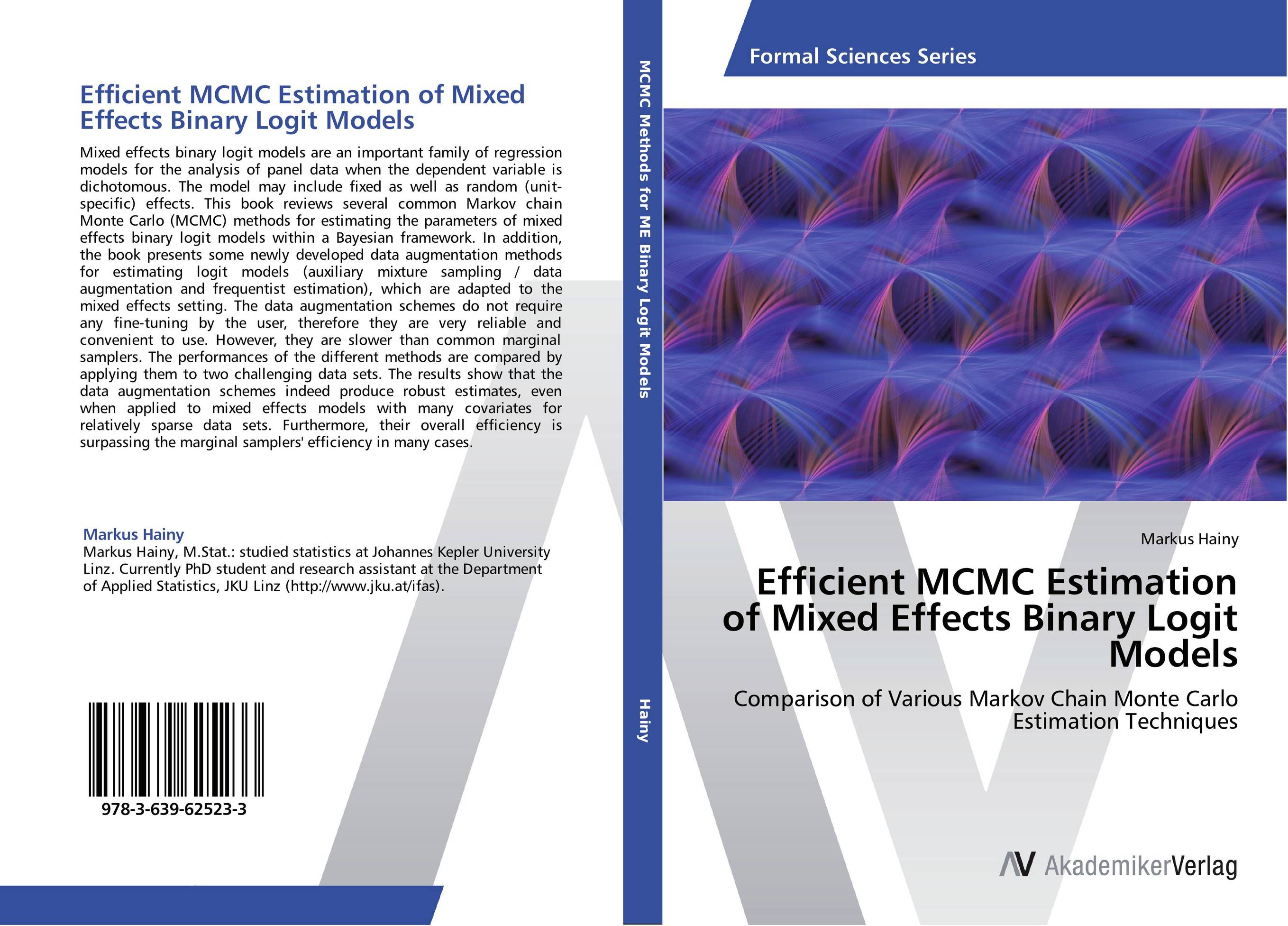
В наличии
Местонахождение: Алматы | Состояние экземпляра: новый |

Бумажная
версия
версия
Автор: Markus Hainy
ISBN: 9783639625233
Год издания: 2014
Формат книги: 60×90/16 (145×215 мм)
Количество страниц: 164
Издательство: AV Akademikerverlag
Цена: 27870 тг
Положить в корзину
Способы доставки в город Алматы * комплектация (срок до отгрузки) не более 2 рабочих дней |
Самовывоз из города Алматы (пункты самовывоза партнёра CDEK) |
Курьерская доставка CDEK из города Москва |
Доставка Почтой России из города Москва |
Аннотация: Mixed effects binary logit models are an important family of regression models for the analysis of panel data when the dependent variable is dichotomous. The model may include fixed as well as random (unit-specific) effects. This book reviews several common Markov chain Monte Carlo (MCMC) methods for estimating the parameters of mixed effects binary logit models within a Bayesian framework. In addition, the book presents some newly developed data augmentation methods for estimating logit models (auxiliary mixture sampling / data augmentation and frequentist estimation), which are adapted to the mixed effects setting. The data augmentation schemes do not require any fine-tuning by the user, therefore they are very reliable and convenient to use. However, they are slower than common marginal samplers. The performances of the different methods are compared by applying them to two challenging data sets. The results show that the data augmentation schemes indeed produce robust estimates, even when applied to mixed effects models with many covariates for relatively sparse data sets. Furthermore, their overall efficiency is surpassing the marginal samplers' efficiency in many cases.
Ключевые слова: binary, Binary Data, augmentation, logit, mixed effects, MCMC, Markov, Monte Carlo