Поиск по каталогу |
(строгое соответствие)
|
- Профессиональная
- Научно-популярная
- Художественная
- Публицистика
- Детская
- Искусство
- Хобби, семья, дом
- Спорт
- Путеводители
- Блокноты, тетради, открытки
Effective Dimensionality Reduction in Pattern Recognition.
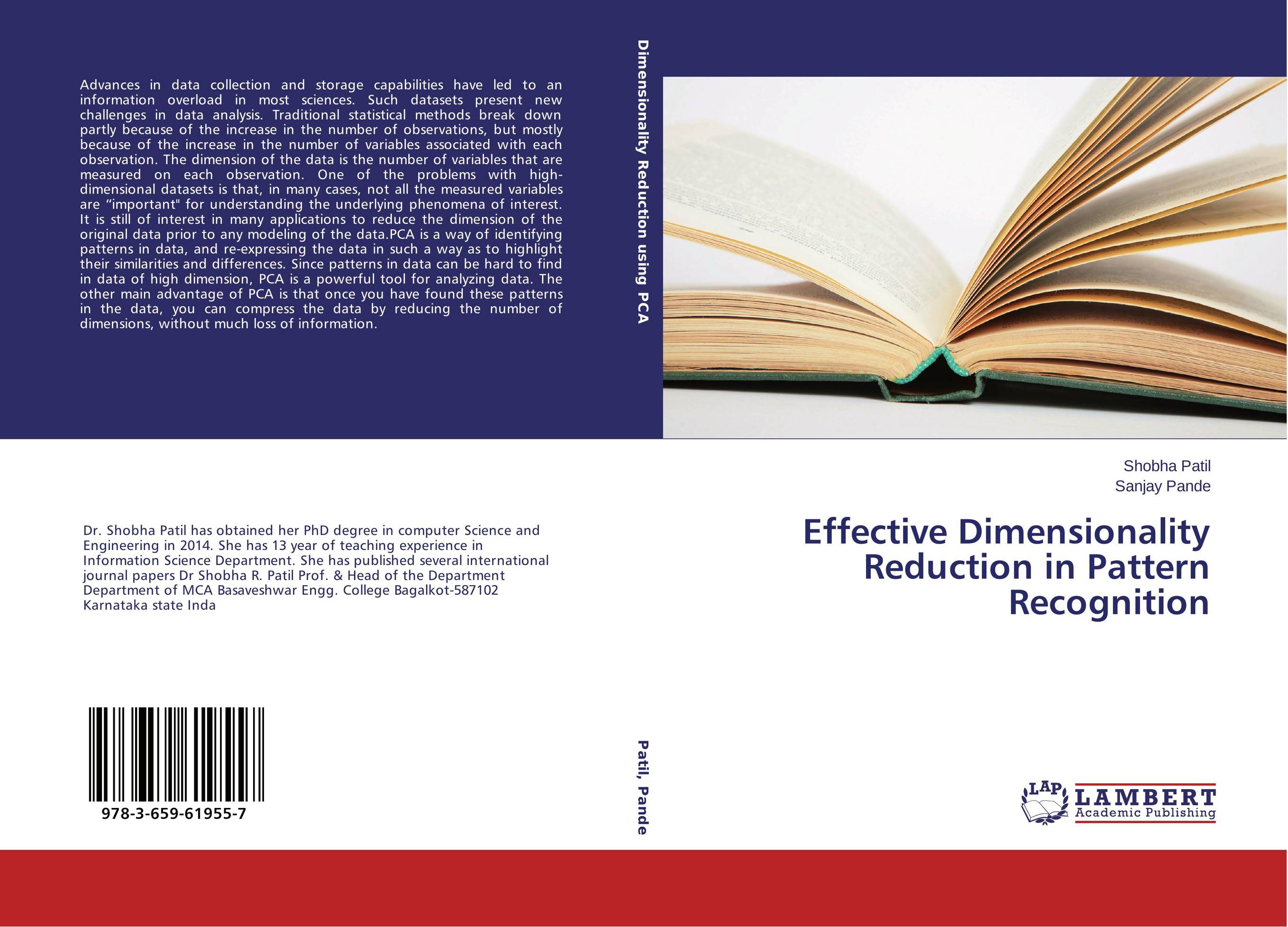
В наличии
Местонахождение: Алматы | Состояние экземпляра: новый |

Бумажная
версия
версия
Автор: Shobha Patil and Sanjay Pande
ISBN: 9783659619557
Год издания: 2014
Формат книги: 60×90/16 (145×215 мм)
Количество страниц: 160
Издательство: LAP LAMBERT Academic Publishing
Цена: 42249 тг
Положить в корзину
Способы доставки в город Алматы * комплектация (срок до отгрузки) не более 2 рабочих дней |
Самовывоз из города Алматы (пункты самовывоза партнёра CDEK) |
Курьерская доставка CDEK из города Москва |
Доставка Почтой России из города Москва |
Аннотация: Advances in data collection and storage capabilities have led to an information overload in most sciences. Such datasets present new challenges in data analysis. Traditional statistical methods break down partly because of the increase in the number of observations, but mostly because of the increase in the number of variables associated with each observation. The dimension of the data is the number of variables that are measured on each observation. One of the problems with high-dimensional datasets is that, in many cases, not all the measured variables are “important" for understanding the underlying phenomena of interest. It is still of interest in many applications to reduce the dimension of the original data prior to any modeling of the data.PCA is a way of identifying patterns in data, and re-expressing the data in such a way as to highlight their similarities and differences. Since patterns in data can be hard to find in data of high dimension, PCA is a powerful tool for analyzing data. The other main advantage of PCA is that once you have found these patterns in the data, you can compress the data by reducing the number of dimensions, without much loss of information.
Ключевые слова: Face recognition, PCA, Dimensionality Reduction, NEURAL NETWORKS., Euclidean Distance Classifire