Поиск по каталогу |
(строгое соответствие)
|
- Профессиональная
- Научно-популярная
- Художественная
- Публицистика
- Детская
- Искусство
- Хобби, семья, дом
- Спорт
- Путеводители
- Блокноты, тетради, открытки
Agent-based models and machine learning in decision support systems. Application to resource allocation in situations of urban catastrophes
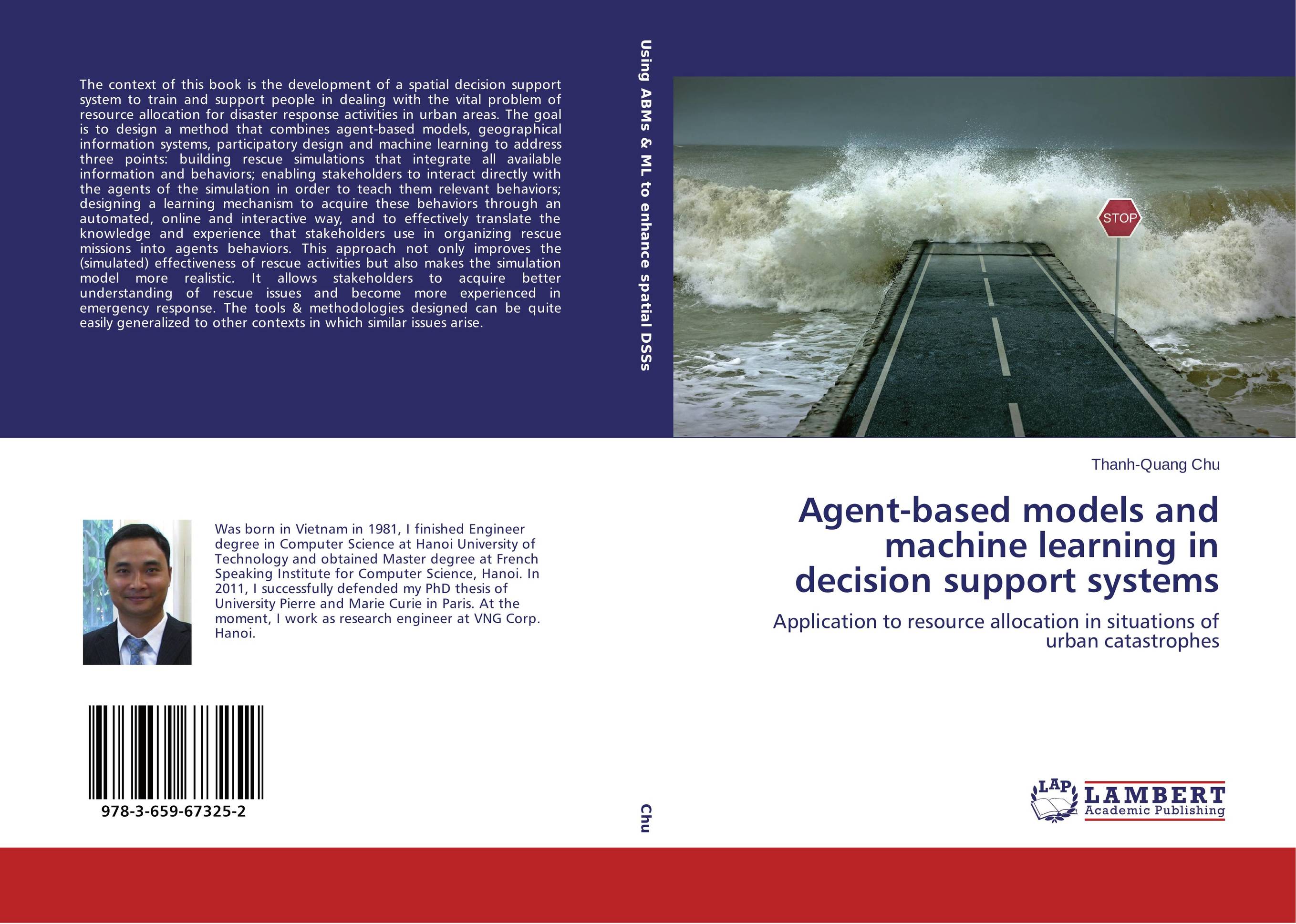
В наличии
Местонахождение: Алматы | Состояние экземпляра: новый |

Бумажная
версия
версия
Автор: Thanh-Quang Chu
ISBN: 9783659673252
Год издания: 2015
Формат книги: 60×90/16 (145×215 мм)
Количество страниц: 208
Издательство: LAP LAMBERT Academic Publishing
Цена: 43954 тг
Положить в корзину
Позиции в рубрикаторе
Отрасли знаний:Код товара: 143334
Способы доставки в город Алматы * комплектация (срок до отгрузки) не более 2 рабочих дней |
Самовывоз из города Алматы (пункты самовывоза партнёра CDEK) |
Курьерская доставка CDEK из города Москва |
Доставка Почтой России из города Москва |
Аннотация: The context of this book is the development of a spatial decision support system to train and support people in dealing with the vital problem of resource allocation for disaster response activities in urban areas. The goal is to design a method that combines agent-based models, geographical information systems, participatory design and machine learning to address three points: building rescue simulations that integrate all available information and behaviors; enabling stakeholders to interact directly with the agents of the simulation in order to teach them relevant behaviors; designing a learning mechanism to acquire these behaviors through an automated, online and interactive way, and to effectively translate the knowledge and experience that stakeholders use in organizing rescue missions into agents behaviors. This approach not only improves the (simulated) effectiveness of rescue activities but also makes the simulation model more realistic. It allows stakeholders to acquire better understanding of rescue issues and become more experienced in emergency response. The tools & methodologies designed can be quite easily generalized to other contexts in which similar issues arise.
Ключевые слова: Agent-Based Modeling, disaster response, Emergency Management, Machine Learning, participatory design, Utility Function, Spatial Decision Support System, Agent-Based Modeling, disaster response, Emergency Management, Machine Learning, participatory design, Utility Function, Spatial Decision Support System, Agent-Based Modeling, Decision Tree, disaster response, Emergency Management, Geographical Information System, knowledge extraction, Machine Learning, multi-agent system, participatory design, Utility Function, Spatial Decision Support System, Role-Playing Game, Agent-Based Participatory Simulation, Preference Elicitation