Поиск по каталогу |
(строгое соответствие)
|
- Профессиональная
- Научно-популярная
- Художественная
- Публицистика
- Детская
- Искусство
- Хобби, семья, дом
- Спорт
- Путеводители
- Блокноты, тетради, открытки
Time Series Forecasting Using Statistical And Neural Networks Models.
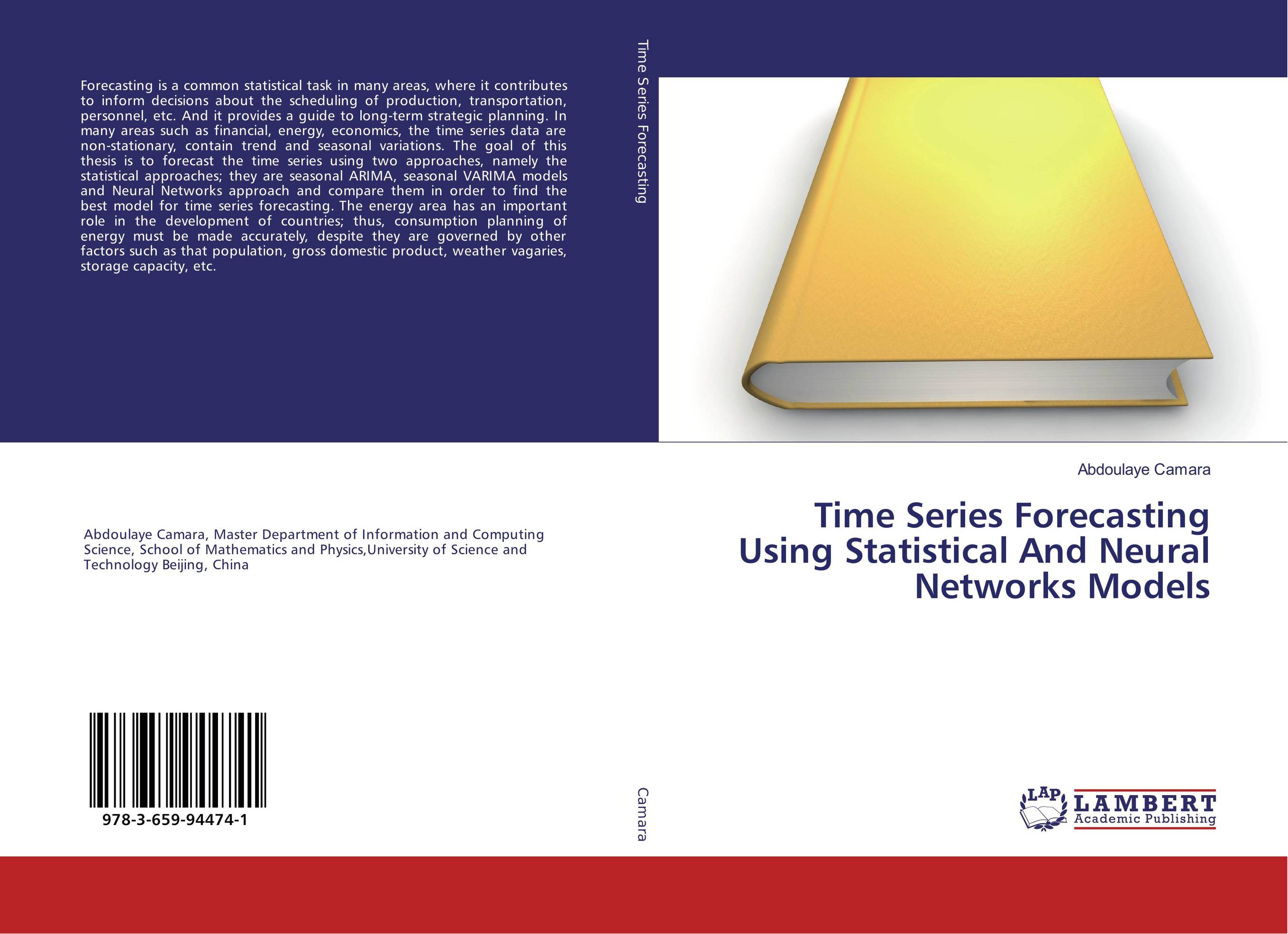
В наличии
Местонахождение: Алматы | Состояние экземпляра: новый |

Бумажная
версия
версия
Автор: Abdoulaye Camara
ISBN: 9783659944741
Год издания: 2016
Формат книги: 60×90/16 (145×215 мм)
Количество страниц: 120
Издательство: LAP LAMBERT Academic Publishing
Цена: 30180 тг
Положить в корзину
Способы доставки в город Алматы * комплектация (срок до отгрузки) не более 2 рабочих дней |
Самовывоз из города Алматы (пункты самовывоза партнёра CDEK) |
Курьерская доставка CDEK из города Москва |
Доставка Почтой России из города Москва |
Аннотация: Forecasting is a common statistical task in many areas, where it contributes to inform decisions about the scheduling of production, transportation, personnel, etc. And it provides a guide to long-term strategic planning. In many areas such as financial, energy, economics, the time series data are non-stationary, contain trend and seasonal variations. The goal of this thesis is to forecast the time series using two approaches, namely the statistical approaches; they are seasonal ARIMA, seasonal VARIMA models and Neural Networks approach and compare them in order to find the best model for time series forecasting. The energy area has an important role in the development of countries; thus, consumption planning of energy must be made accurately, despite they are governed by other factors such as that population, gross domestic product, weather vagaries, storage capacity, etc.
Ключевые слова: ARIMA Models, seasonality, Time series forecasting, Feedforward Neural Networks, VARIMA Models
Похожие издания
![]() | Отрасли знаний: Точные науки -> Информатика и программирование Abhishek Das and Samit Bhanja Time Series Forecasting using Deep Learning. . 2020 г., 52 стр., мягкий переплет Deep Learning which comprises Deep Neural Networks (DNNs) has achieved excellent success in image classification, speech recognition, etc. But DNNs suffer a lot of challenges for time series forecasting (TSF) because most of the time-series data are nonlinear in nature and highly dynamic in behavior. TSF has a great impact on our socio-economic... | 22924 тг ![]() |
![]() | Отрасли экономики: Промышленность в целом Swati Sharma and Vinod Kumar Neural Network and Fuzzy Time Series. Forecasting using neural network and fuzzy time series. 2019 г., 88 стр., мягкий переплет This work deals with neural networks (NN), specifically with multi-layered NN from the algorithm learning point of view. We will describe feed forward neural network (FFNN), recurrent neural network (RCNN) and introduce basic facts about NN, which will be used later in dissertation. A neural network is a mathematical model that is inspired by... | 31463 тг ![]() |
![]() | Отрасли знаний: Точные науки -> Математика -> Статистика ?zge Tu?rul S?nmez Methods for Time Series Forecasting. With Applications in R. 2017 г., 264 стр., мягкий переплет This book provides a comparison of different forecasting methods with special implementations for different time series data and evaluates the results of forecasting experiments. It is important for the selected forecasting method to give more accurate results and smaller forecast errors. Also, the coding and the implementation processes have an... | 47394 тг ![]() |
![]() | Отрасли знаний: Точные науки -> Математика Arnaud Trebaol High Frequency Time Series Forecasting. . 2015 г., 160 стр., мягкий переплет Time series are a special form of data where past values in the series may influence future values, depending on the presence of underlying deterministic forces. These forces may be characterised by trends, cycles and nonstationary behaviour in the time series and predictive models attempt to recognise the recurring patterns and more particularly... | 42249 тг ![]() |