Поиск по каталогу |
(строгое соответствие)
|
- Профессиональная
- Научно-популярная
- Художественная
- Публицистика
- Детская
- Искусство
- Хобби, семья, дом
- Спорт
- Путеводители
- Блокноты, тетради, открытки
Forecasting with Many Predictors. Complete Subset Regression Versus Shrinkage Methods and Extreme Gradient Boosting
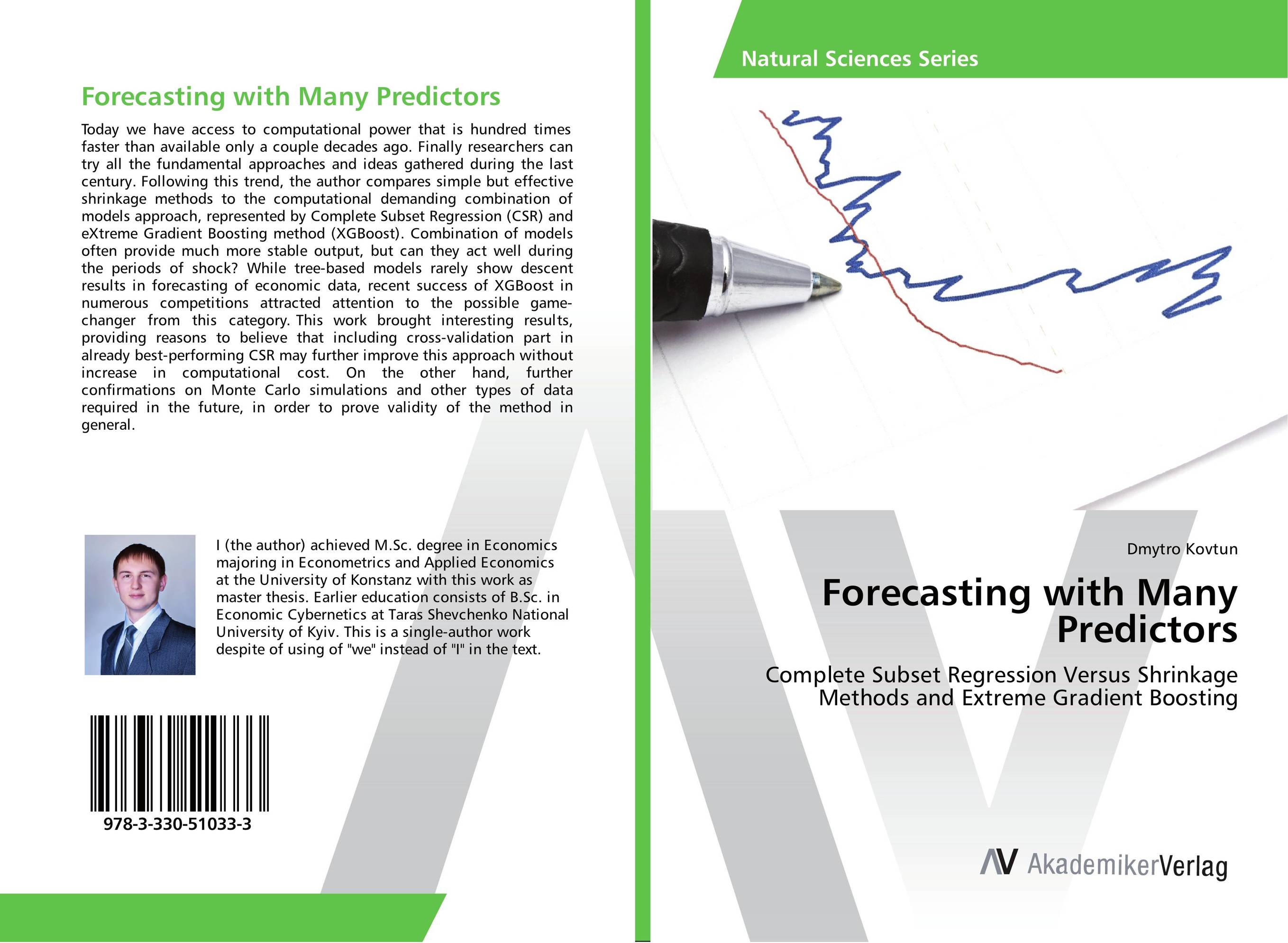
В наличии
Местонахождение: Алматы | Состояние экземпляра: новый |

Бумажная
версия
версия
Автор: Dmytro Kovtun
ISBN: 9783330510333
Год издания: 2016
Формат книги: 60×90/16 (145×215 мм)
Количество страниц: 64
Издательство: AV Akademikerverlag
Цена: 18027 тг
Положить в корзину
Позиции в рубрикаторе
Сферы деятельности:Код товара: 166022
Способы доставки в город Алматы * комплектация (срок до отгрузки) не более 2 рабочих дней |
Самовывоз из города Алматы (пункты самовывоза партнёра CDEK) |
Курьерская доставка CDEK из города Москва |
Доставка Почтой России из города Москва |
Аннотация: Today we have access to computational power that is hundred times faster than available only a couple decades ago. Finally researchers can try all the fundamental approaches and ideas gathered during the last century. Following this trend, the author compares simple but effective shrinkage methods to the computational demanding combination of models approach, represented by Complete Subset Regression (CSR) and eXtreme Gradient Boosting method (XGBoost). Combination of models often provide much more stable output, but can they act well during the periods of shock? While tree-based models rarely show descent results in forecasting of economic data, recent success of XGBoost in numerous competitions attracted attention to the possible game-changer from this category. This work brought interesting results, providing reasons to believe that including cross-validation part in already best-performing CSR may further improve this approach without increase in computational cost. On the other hand, further confirmations on Monte Carlo simulations and other types of data required in the future, in order to prove validity of the method in general.
Ключевые слова: Econometrics, complete subset regression, combination of models, shrinkage methods, extreme gradient boosting