Поиск по каталогу |
(строгое соответствие)
|
- Профессиональная
- Научно-популярная
- Художественная
- Публицистика
- Детская
- Искусство
- Хобби, семья, дом
- Спорт
- Путеводители
- Блокноты, тетради, открытки
Analyzing Student Performance Prediction: Meta Stacking Classification.
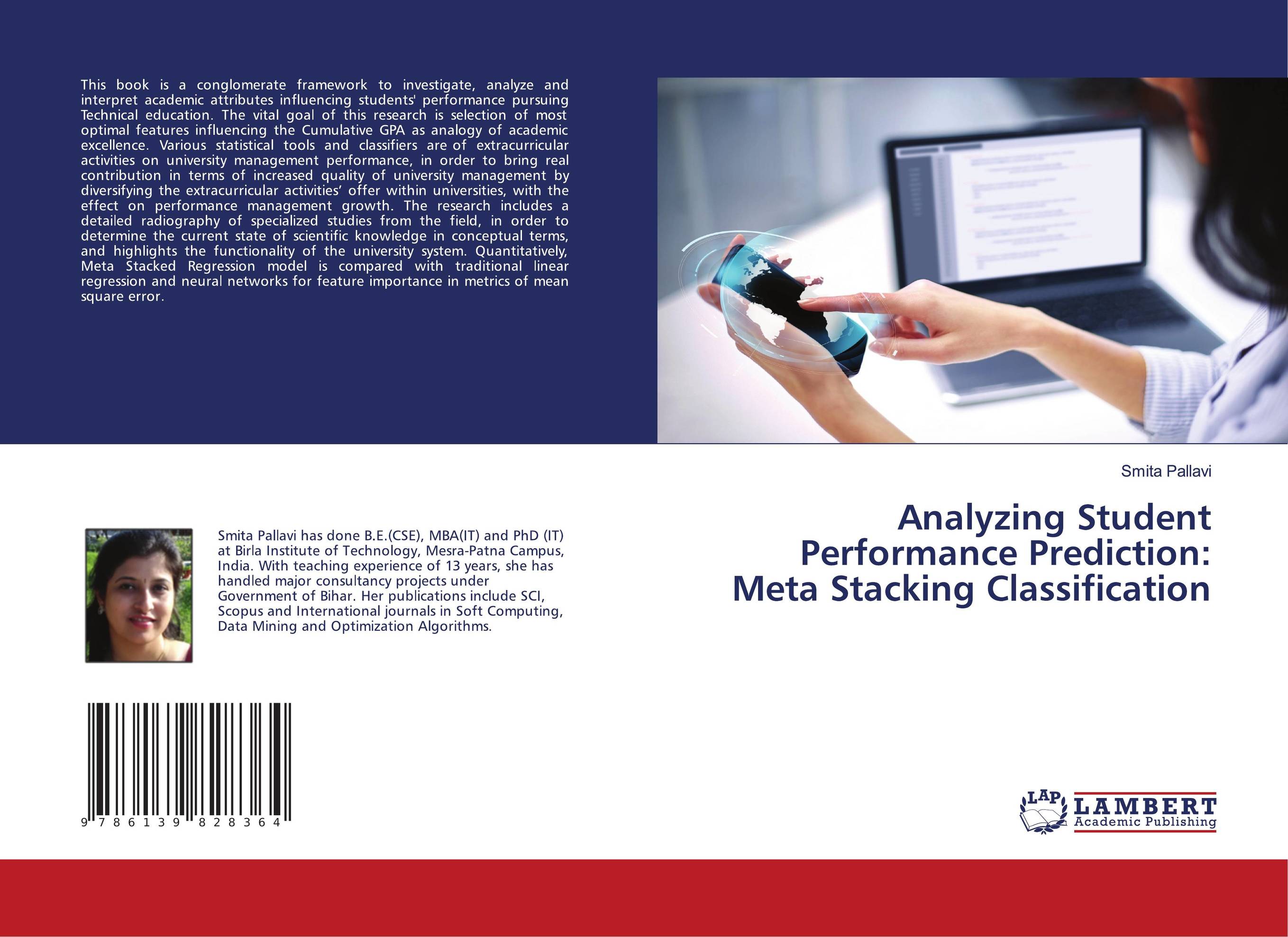
В наличии
Местонахождение: Алматы | Состояние экземпляра: новый |

Бумажная
версия
версия
Автор: Smita Pallavi
ISBN: 9786139828364
Год издания: 2018
Формат книги: 60×90/16 (145×215 мм)
Количество страниц: 104
Издательство: LAP LAMBERT Academic Publishing
Цена: 29611 тг
Положить в корзину
Способы доставки в город Алматы * комплектация (срок до отгрузки) не более 2 рабочих дней |
Самовывоз из города Алматы (пункты самовывоза партнёра CDEK) |
Курьерская доставка CDEK из города Москва |
Доставка Почтой России из города Москва |
Аннотация: This book is a conglomerate framework to investigate, analyze and interpret academic attributes influencing students' performance pursuing Technical education. The vital goal of this research is selection of most optimal features influencing the Cumulative GPA as analogy of academic excellence. Various statistical tools and classifiers are of extracurricular activities on university management performance, in order to bring real contribution in terms of increased quality of university management by diversifying the extracurricular activities’ offer within universities, with the effect on performance management growth. The research includes a detailed radiography of specialized studies from the field, in order to determine the current state of scientific knowledge in conceptual terms, and highlights the functionality of the university system. Quantitatively, Meta Stacked Regression model is compared with traditional linear regression and neural networks for feature importance in metrics of mean square error.
Ключевые слова: Academic Excellence, neural networks, regression, Nature inspired Optimization, Student decision making