Поиск по каталогу |
(строгое соответствие)
|
- Профессиональная
- Научно-популярная
- Художественная
- Публицистика
- Детская
- Искусство
- Хобби, семья, дом
- Спорт
- Путеводители
- Блокноты, тетради, открытки
Mining Sequential Rules By Applying Sliding Window Constraint. Mining of Partially-Ordered Sequential Rules (POSR) By Applying the Sliding-Window Constraint
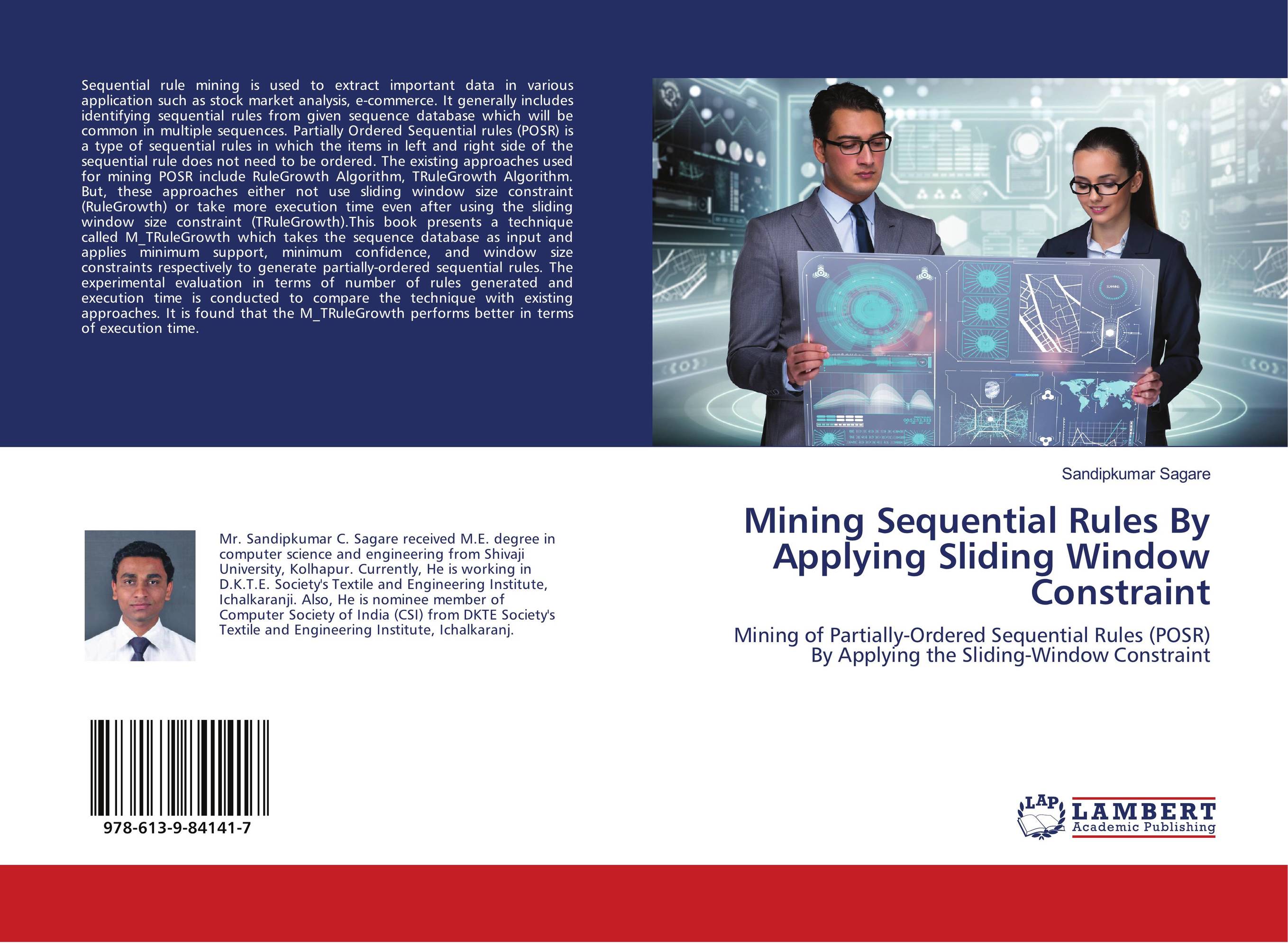
В наличии
Местонахождение: Алматы | Состояние экземпляра: новый |

Бумажная
версия
версия
Автор: Sandipkumar Sagare
ISBN: 9786139841417
Год издания: 2018
Формат книги: 60×90/16 (145×215 мм)
Количество страниц: 64
Издательство: LAP LAMBERT Academic Publishing
Цена: 21414 тг
Положить в корзину
Способы доставки в город Алматы * комплектация (срок до отгрузки) не более 2 рабочих дней |
Самовывоз из города Алматы (пункты самовывоза партнёра CDEK) |
Курьерская доставка CDEK из города Москва |
Доставка Почтой России из города Москва |
Аннотация: Sequential rule mining is used to extract important data in various application such as stock market analysis, e-commerce. It generally includes identifying sequential rules from given sequence database which will be common in multiple sequences. Partially Ordered Sequential rules (POSR) is a type of sequential rules in which the items in left and right side of the sequential rule does not need to be ordered. The existing approaches used for mining POSR include RuleGrowth Algorithm, TRuleGrowth Algorithm. But, these approaches either not use sliding window size constraint (RuleGrowth) or take more execution time even after using the sliding window size constraint (TRuleGrowth).This book presents a technique called M_TRuleGrowth which takes the sequence database as input and applies minimum support, minimum confidence, and window size constraints respectively to generate partially-ordered sequential rules. The experimental evaluation in terms of number of rules generated and execution time is conducted to compare the technique with existing approaches. It is found that the M_TRuleGrowth performs better in terms of execution time.
Ключевые слова: association rule, sequence, Sequential Pattern mining, Sequential Rule, Sequential Rule mining