Поиск по каталогу |
(строгое соответствие)
|
- Профессиональная
- Научно-популярная
- Художественная
- Публицистика
- Детская
- Искусство
- Хобби, семья, дом
- Спорт
- Путеводители
- Блокноты, тетради, открытки
Correlation Structure-Property in the case of Odorant Molecules. QSPR study and Molecular Docking
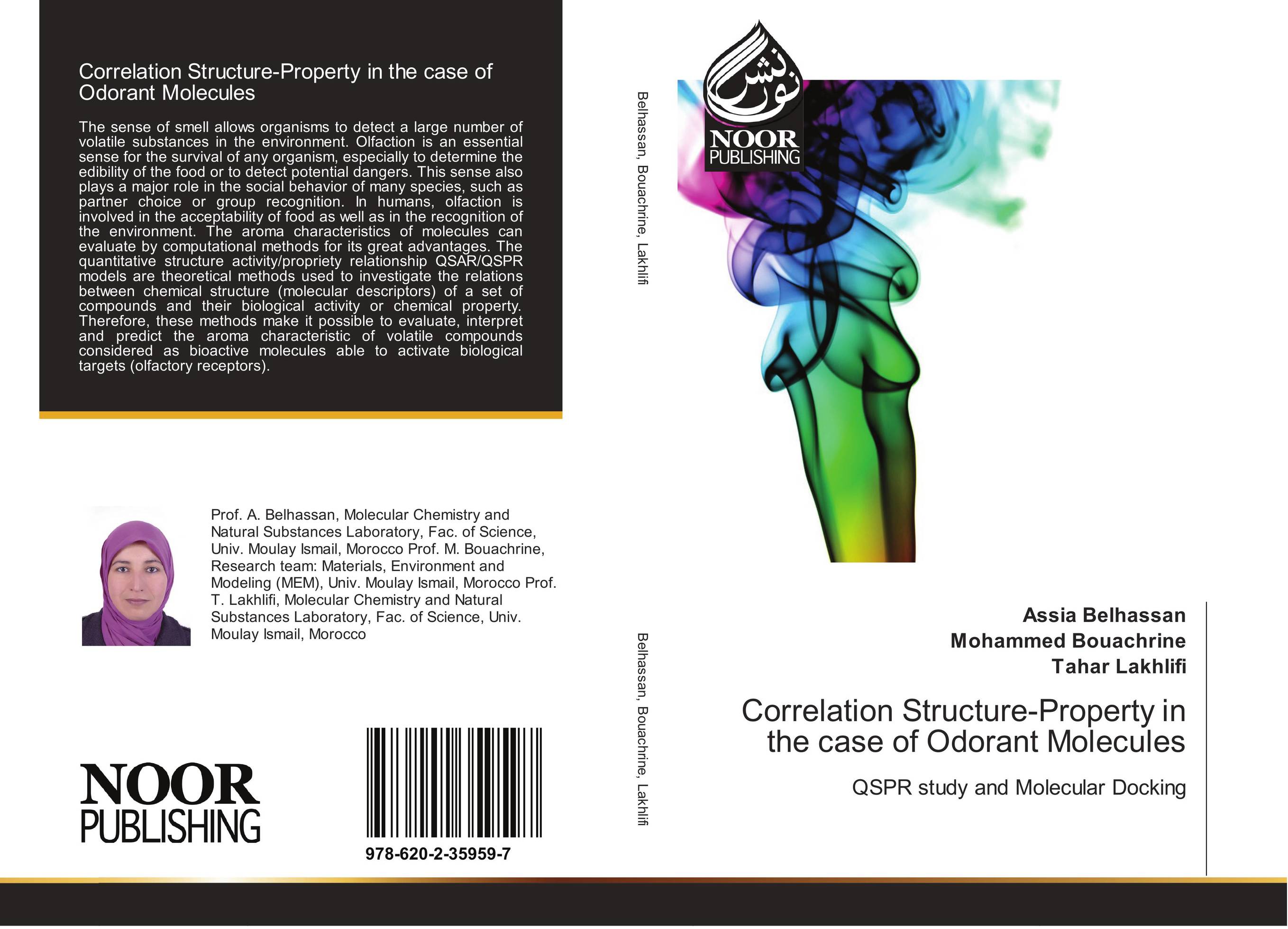
В наличии
Местонахождение: Алматы | Состояние экземпляра: новый |

Бумажная
версия
версия
Автор: Assia Belhassan,Mohammed Bouachrine and Tahar Lakhlifi
ISBN: 9786202359597
Год издания: 2019
Формат книги: 60×90/16 (145×215 мм)
Количество страниц: 164
Издательство: Noor Publishing
Цена: 27870 тг
Положить в корзину
Способы доставки в город Алматы * комплектация (срок до отгрузки) не более 2 рабочих дней |
Самовывоз из города Алматы (пункты самовывоза партнёра CDEK) |
Курьерская доставка CDEK из города Москва |
Доставка Почтой России из города Москва |
Аннотация: The sense of smell allows organisms to detect a large number of volatile substances in the environment. Olfaction is an essential sense for the survival of any organism, especially to determine the edibility of the food or to detect potential dangers. This sense also plays a major role in the social behavior of many species, such as partner choice or group recognition. In humans, olfaction is involved in the acceptability of food as well as in the recognition of the environment. The aroma characteristics of molecules can evaluate by computational methods for its great advantages. The quantitative structure activity/propriety relationship QSAR/QSPR models are theoretical methods used to investigate the relations between chemical structure (molecular descriptors) of a set of compounds and their biological activity or chemical property. Therefore, these methods make it possible to evaluate, interpret and predict the aroma characteristic of volatile compounds considered as bioactive molecules able to activate biological targets (olfactory receptors).
Ключевые слова: Odorant molecules, Quantitative Structure Property Relationship, Multiple Linear Regression, Artificial Neural Network