Поиск по каталогу |
(строгое соответствие)
|
- Профессиональная
- Научно-популярная
- Художественная
- Публицистика
- Детская
- Искусство
- Хобби, семья, дом
- Спорт
- Путеводители
- Блокноты, тетради, открытки
BrainAGE. A novel machine learning approach for identifying abnormal age-related brain changes
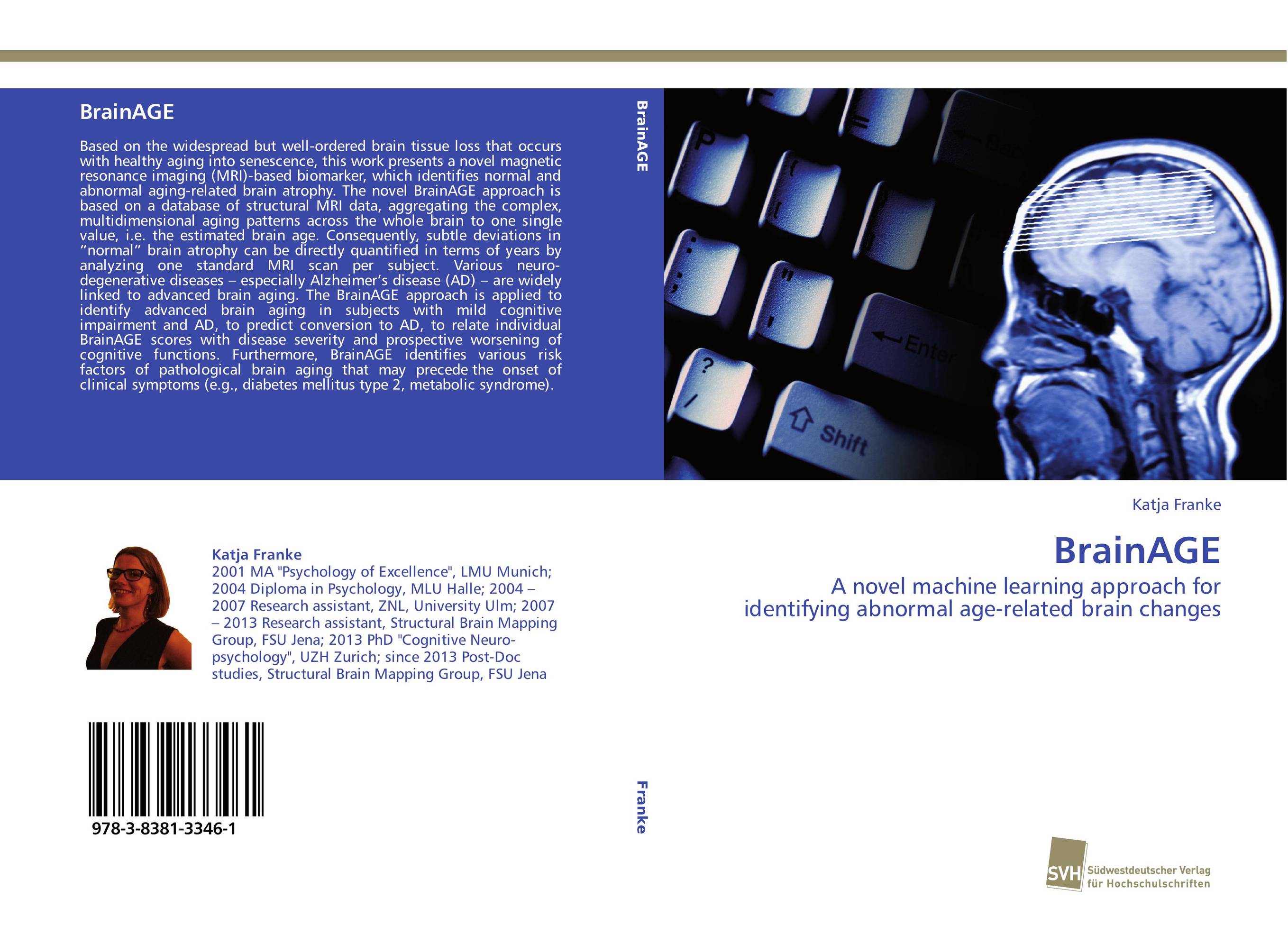
В наличии
Местонахождение: Алматы | Состояние экземпляра: новый |

Бумажная
версия
версия
Автор: Katja Franke
ISBN: 9783838133461
Год издания: 2014
Формат книги: 60×90/16 (145×215 мм)
Количество страниц: 168
Издательство: S?dwestdeutscher Verlag f?r Hochschulschriften
Цена: 38661 тг
Положить в корзину
Способы доставки в город Алматы * комплектация (срок до отгрузки) не более 2 рабочих дней |
Самовывоз из города Алматы (пункты самовывоза партнёра CDEK) |
Курьерская доставка CDEK из города Москва |
Доставка Почтой России из города Москва |
Аннотация: Based on the widespread but well-ordered brain tissue loss that occurs with healthy aging into senescence, this work presents a novel magnetic resonance imaging (MRI)-based biomarker, which identifies normal and abnormal aging-related brain atrophy. The novel BrainAGE approach is based on a database of structural MRI data, aggregating the complex, multidimensional aging patterns across the whole brain to one single value, i.e. the estimated brain age. Consequently, subtle deviations in “normal” brain atrophy can be directly quantified in terms of years by analyzing one standard MRI scan per subject. Various neuro-degenerative diseases – especially Alzheimer’s disease (AD) – are widely linked to advanced brain aging. The BrainAGE approach is applied to identify advanced brain aging in subjects with mild cognitive impairment and AD, to predict conversion to AD, to relate individual BrainAGE scores with disease severity and prospective worsening of cognitive functions. Furthermore, BrainAGE identifies various risk factors of pathological brain aging that may precede the onset of clinical symptoms (e.g., diabetes mellitus type 2, metabolic syndrome).
Ключевые слова: machine learning, mustererkennung, Alzheimer Demenz, Neurowissenschaft, strukturelles MRT, Relevance vector regression, Gehirnentwicklung, Gehirnalterung