Поиск по каталогу |
(строгое соответствие)
|
- Профессиональная
- Научно-популярная
- Художественная
- Публицистика
- Детская
- Искусство
- Хобби, семья, дом
- Спорт
- Путеводители
- Блокноты, тетради, открытки
Customer Portfolio of a consumer goods based virtual store. Identifying customer segments with Cluster Analysis
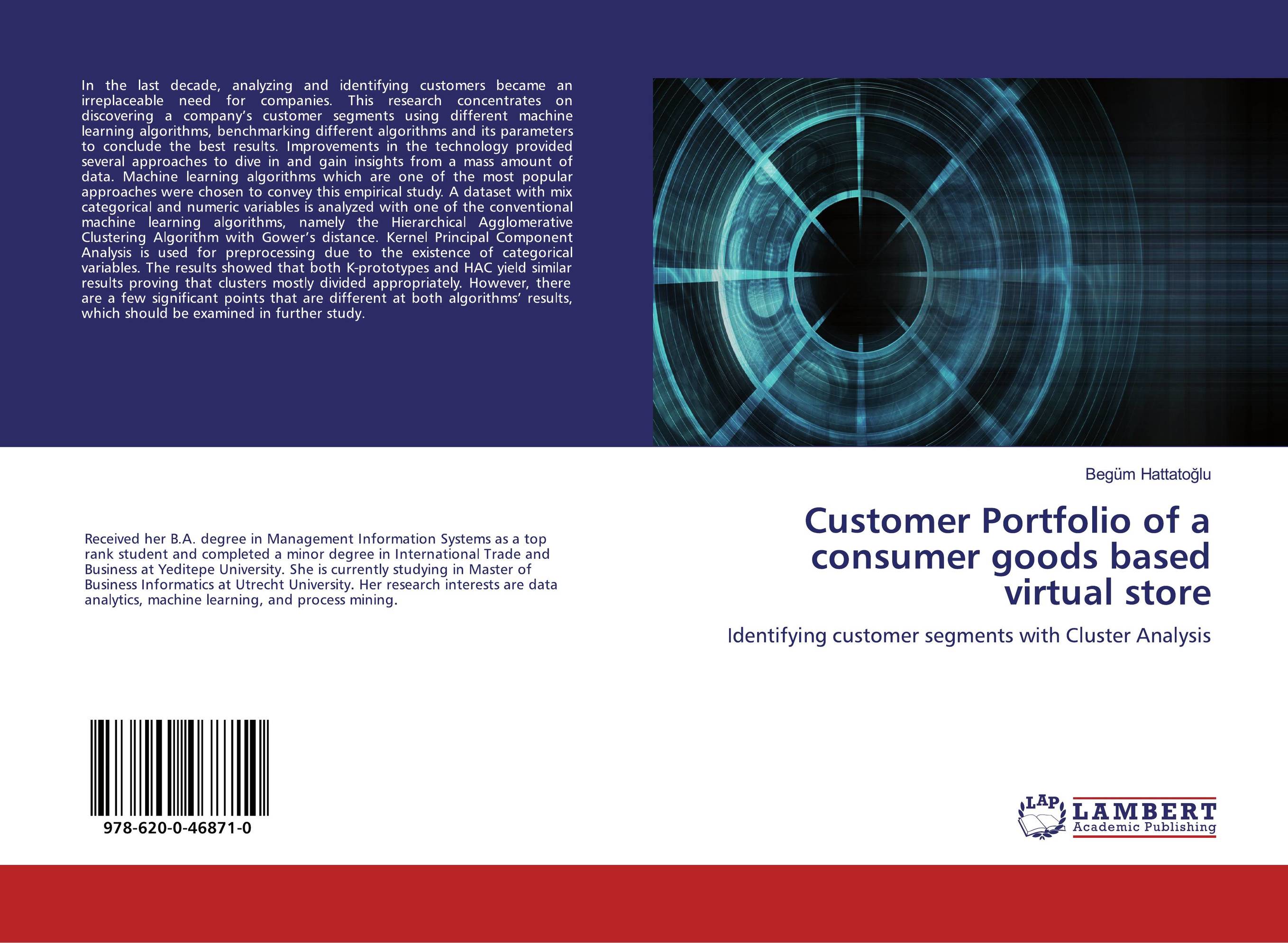
В наличии
Местонахождение: Алматы | Состояние экземпляра: новый |

Бумажная
версия
версия
Автор: Beg?m Hattato?lu
ISBN: 9786200468710
Год издания: 2019
Формат книги: 60×90/16 (145×215 мм)
Количество страниц: 68
Издательство: LAP LAMBERT Academic Publishing
Цена: 23493 тг
Положить в корзину
Позиции в рубрикаторе
Сферы деятельности:Код товара: 506623
Способы доставки в город Алматы * комплектация (срок до отгрузки) не более 2 рабочих дней |
Самовывоз из города Алматы (пункты самовывоза партнёра CDEK) |
Курьерская доставка CDEK из города Москва |
Доставка Почтой России из города Москва |
Аннотация: In the last decade, analyzing and identifying customers became an irreplaceable need for companies. This research concentrates on discovering a company’s customer segments using different machine learning algorithms, benchmarking different algorithms and its parameters to conclude the best results. Improvements in the technology provided several approaches to dive in and gain insights from a mass amount of data. Machine learning algorithms which are one of the most popular approaches were chosen to convey this empirical study. A dataset with mix categorical and numeric variables is analyzed with one of the conventional machine learning algorithms, namely the Hierarchical Agglomerative Clustering Algorithm with Gower’s distance. Kernel Principal Component Analysis is used for preprocessing due to the existence of categorical variables. The results showed that both K-prototypes and HAC yield similar results proving that clusters mostly divided appropriately. However, there are a few significant points that are different at both algorithms’ results, which should be examined in further study.
Ключевые слова: Machine Learning, Kernel Principal Component Analysis, Clustering, Hierarchical Agglomerative Clustering, Gower’s Distance, K-prototypes