Поиск по каталогу |
(строгое соответствие)
|
- Профессиональная
- Научно-популярная
- Художественная
- Публицистика
- Детская
- Искусство
- Хобби, семья, дом
- Спорт
- Путеводители
- Блокноты, тетради, открытки
Accurately Forecasting Stock Prices using LSTM and GRU Neural Networks. A Deep Learning approach for forecasting stock price time-series data in groups
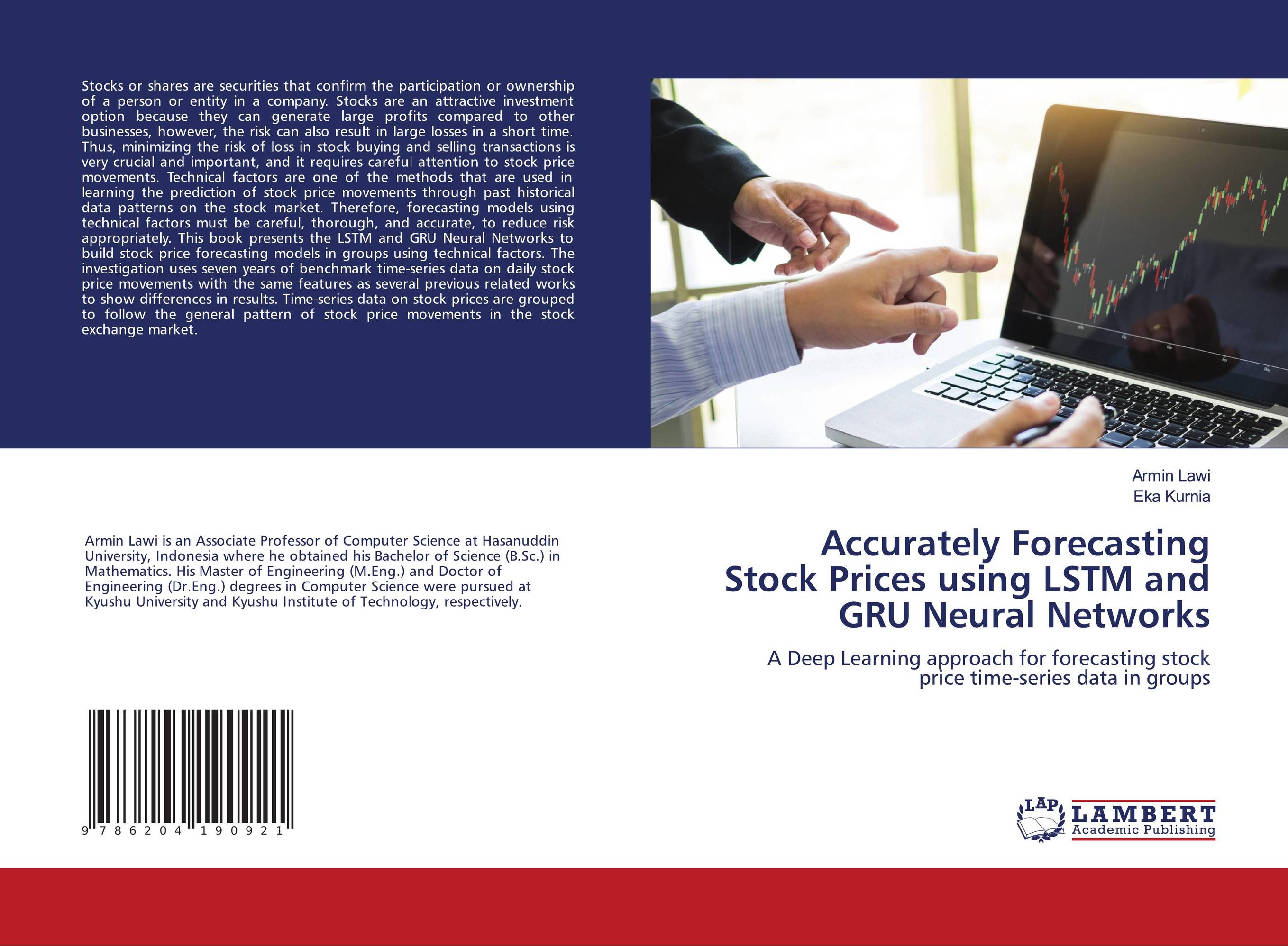
В наличии
Местонахождение: Алматы | Состояние экземпляра: новый |

Бумажная
версия
версия
Автор: Armin Lawi and Eka Kurnia
ISBN: 9786204190921
Год издания: 1905
Формат книги: 60×90/16 (145×215 мм)
Количество страниц: 52
Издательство: LAP LAMBERT Academic Publishing
Цена: 22924 тг
Положить в корзину
Позиции в рубрикаторе
Сферы деятельности:Код товара: 714095
Способы доставки в город Алматы * комплектация (срок до отгрузки) не более 2 рабочих дней |
Самовывоз из города Алматы (пункты самовывоза партнёра CDEK) |
Курьерская доставка CDEK из города Москва |
Доставка Почтой России из города Москва |
Аннотация: Stocks or shares are securities that confirm the participation or ownership of a person or entity in a company. Stocks are an attractive investment option because they can generate large profits compared to other businesses, however, the risk can also result in large losses in a short time. Thus, minimizing the risk of loss in stock buying and selling transactions is very crucial and important, and it requires careful attention to stock price movements. Technical factors are one of the methods that are used in learning the prediction of stock price movements through past historical data patterns on the stock market. Therefore, forecasting models using technical factors must be careful, thorough, and accurate, to reduce risk appropriately. This book presents the LSTM and GRU Neural Networks to build stock price forecasting models in groups using technical factors. The investigation uses seven years of benchmark time-series data on daily stock price movements with the same features as several previous related works to show differences in results. Time-series data on stock prices are grouped to follow the general pattern of stock price movements in the stock exchange market.
Ключевые слова: stock price, Forecasting model, Time-Series Data, Deep Learning, Deep Neural Network, Long Short-Term Memory (LSTM), Gated Recurrent Unit (GRU)