Поиск по каталогу |
(строгое соответствие)
|
- Профессиональная
- Научно-популярная
- Художественная
- Публицистика
- Детская
- Искусство
- Хобби, семья, дом
- Спорт
- Путеводители
- Блокноты, тетради, открытки
Handwritten Semitic Language Digit Recognition Using Deep Learning.
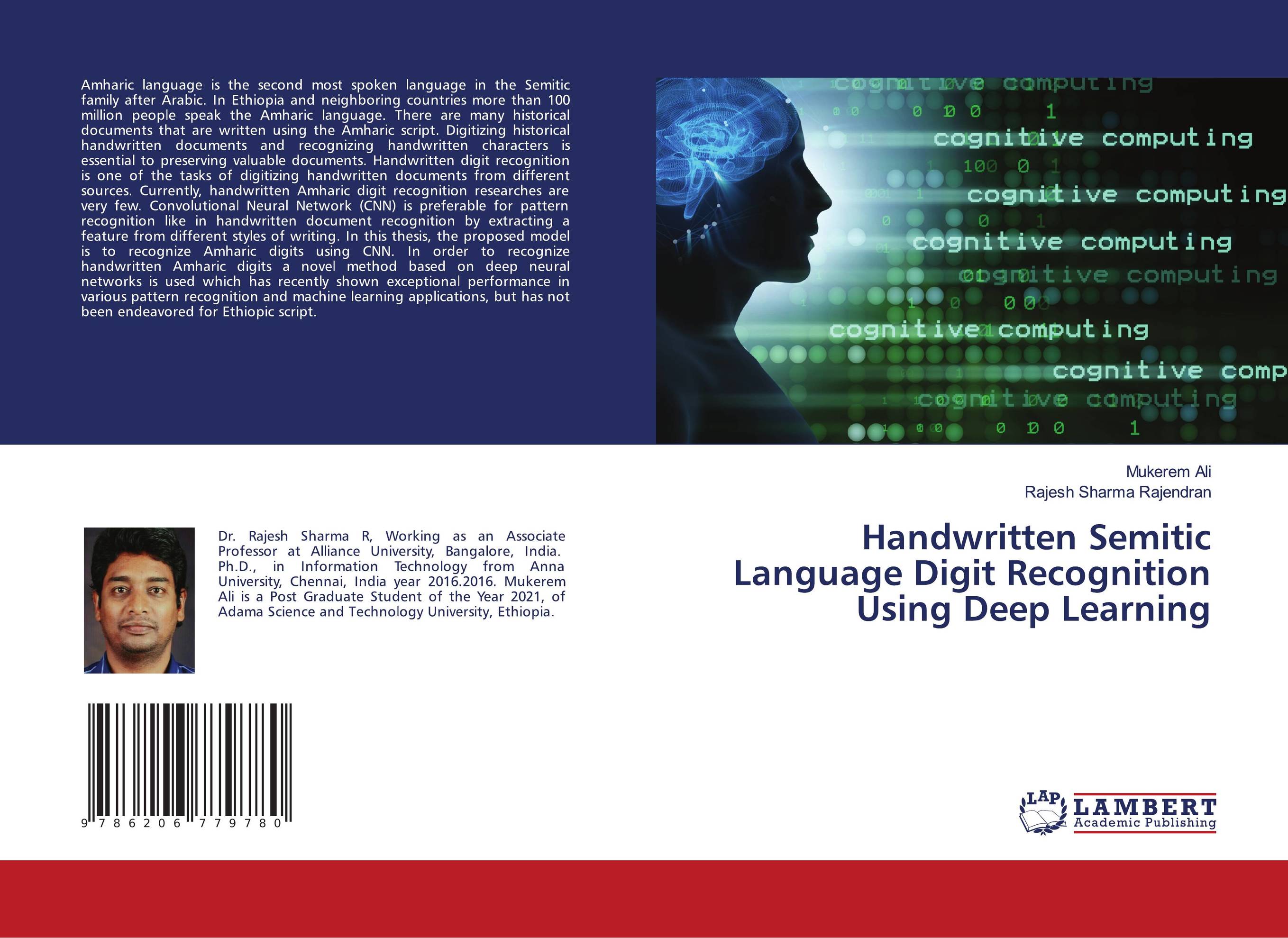
В наличии
Местонахождение: Алматы | Состояние экземпляра: новый |

Бумажная
версия
версия
Автор: Mukerem Ali and Rajesh Sharma Rajendran
ISBN: 9786206779780
Год издания: 1905
Формат книги: 60×90/16 (145×215 мм)
Количество страниц: 96
Издательство: LAP LAMBERT Academic Publishing
Цена: 34651 тг
Положить в корзину
Позиции в рубрикаторе
Сферы деятельности:Код товара: 761940
Способы доставки в город Алматы * комплектация (срок до отгрузки) не более 2 рабочих дней |
Самовывоз из города Алматы (пункты самовывоза партнёра CDEK) |
Курьерская доставка CDEK из города Москва |
Доставка Почтой России из города Москва |
Аннотация: Amharic language is the second most spoken language in the Semitic family after Arabic. In Ethiopia and neighboring countries more than 100 million people speak the Amharic language. There are many historical documents that are written using the Amharic script. Digitizing historical handwritten documents and recognizing handwritten characters is essential to preserving valuable documents. Handwritten digit recognition is one of the tasks of digitizing handwritten documents from different sources. Currently, handwritten Amharic digit recognition researches are very few. Convolutional Neural Network (CNN) is preferable for pattern recognition like in handwritten document recognition by extracting a feature from different styles of writing. In this thesis, the proposed model is to recognize Amharic digits using CNN. In order to recognize handwritten Amharic digits a novel method based on deep neural networks is used which has recently shown exceptional performance in various pattern recognition and machine learning applications, but has not been endeavored for Ethiopic script.
Ключевые слова: CNN, Feature extraction, Digit Recognition, Pattern recognition, Amharic handwritten digit recognition, Deep Learning